Perhaps the most important lesson to be learned with AI/ML is that individual experiments and projects are educational, but they do not lead to business. An organization moving towards scaling AI needs to fundamentally adjust its way of working with AI, in other words, develop its human processes along with its MLOps platform.
From a business perspective, MLOps is the bridge between the experimentation world and the production world: building MLOps means that you’re going beyond AI pilots into a more mature and operationalized way of working with AI. In this blog post, we’ll look into three concrete client cases where we’ve built sustainable AI/ML operations together with the client and conclude with three key steps, that we believe are the three steps of succeeding in creating business value with AI/ML.
From MLOps exploration to an integrated team for global systems and software vendor
Together with the client, we started off by defining the requirements and technology options for a sensitive private cloud environment using f.ex. medical data. The outcome was a customized and standardized approach for machine learning model training automation that enables both consistent ML experimentation in research and AI feature delivery to the end products. The standardized machine learning workflow and tooling brought data scientists and DevOps professionals closer together. This, in turn, enabled them to work towards shared targets and to get from research and PoC phases to product delivery.
Helped a global retail chain to scale AI usage across regions and use cases
We worked with a global consumer brand to enable the data science teams to scale the AI usage across their e-commerce store combining open source technologies with Azure cloud environment. Before Silo AI, the company had already identified use cases for AI – however, the models were built on data science environments isolated from end products. We provided the client team experts that defined and built a model deployment platform that enables AI product teams to deliver the AI-driven e-commerce features in connection to online systems.
From manual machine learning workflows to automated, scalable way-of-working
A data service provider had well-defined MLOps requirements but needed help with making technology choices and building up a solution that would meet their needs for scalable and rapid machine learning model training and reporting. Silo AI experts built a model training infrastructure and automation to speed up their ML workflows using open source tooling and AWS cloud environment. Such a setup also enables deeper collaboration between the data service provider and their clients, as there are better possibilities to accommodate client-specific requirements through such a development environment.
Three steps to take to get from initial pilots to AI at scale
Our Cloud AI & MLOps Tech Area Lead Jukka Remes has been working in a variety of MLOps projects, both in client on-premise and cloud environments. Drawing on his experience, Jukka encapsulates three steps of succeeding in creating business value with AI/ML as follows:
- First, experiment and build a couple of proofs-of-concept to build understanding for going forward.
- Second, after validating your ML approaches and gathering evidence from them, start expanding the involvement of your organization to AI understanding, innovation, development and utilization.
- Third, in order to scale, you’ll need MLOps to address both organizational as well as tooling-related disconnects - bring engineering and data science together and connect them with business through shared framework and standardized practices that accommodate experimentation typical to AI development but also enable productization and business utilization of AI results.
Jukka believes that the key to success with AI is about choosing the battles and focusing on the most value-adding tasks:
“ML development and AI utilization also require a lot of re-organizing, due to the additional complexity that they entail. The most productive tasks are often also the most innovative – meaning they require a lot but give a lot too.”
At the same time, he believes a fully functional MLOps platform is required:
“Having proper MLOps is central in becoming truly able to utilize AI and in growing into an AI-driven organization. The same MLOps framework can be used for turning business problems into developing AI features fluently but also for testing them in practice as a part of the business and the processes and the workflows related to the business.”
About
Want to discuss how Silo AI could help your organization?
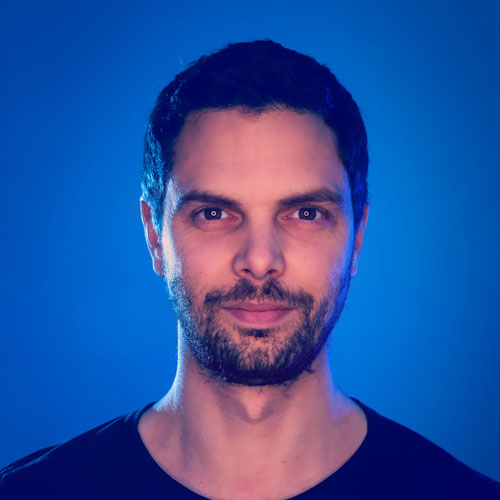
Join the 5000+ subscribers who read the Silo AI monthly newsletter to be among the first to hear about the latest insights, articles, podcast episodes, webinars, and more.