Result
Using the described methodology, we were able to show that companies which reach the maximum risk value in our risk model, during any given quarter, are up to 13 percentage points more likely to experience a stock price decline one month later than the average company.Natural language processing (NLP) as a method has several applications, including uncovering new information from text-based news. In the research Dr. Peter Sarlin and I did, we uncovered how company sentiment in news can be used to signal when a company is at higher probability of stock price decline.
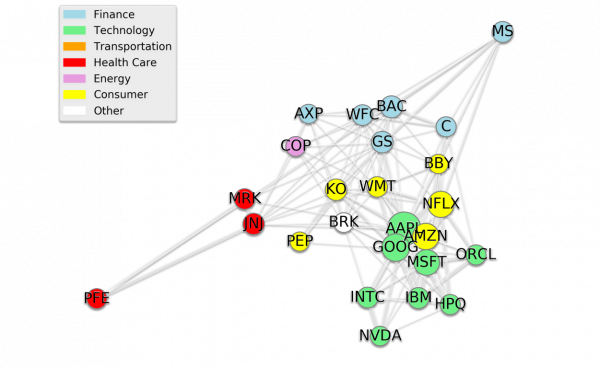
Figure 1: Example network of companies extracted from positive news mentionings.
I have followed stock prices in the US stock market for several years and used news to successfully move in and out of stock and option positions. The idea, developed together with Peter Sarlin, aims at quantifying the precise timing to move in and out of positions, based on how negative the news regarding a company and its peers has recently been.To get started, we needed a dataset of labeled news. One option was to have manually labeled news articles. Another possibility was to extract labels directly from texts that are not labeled using machine learning (example) or using existing APIs like the one provided by Microsoft. We used pre-existing labels (positive or negative) given by the authors of news editorials as this is most likely to represent groundtruth and be a reliable source.We then created networks of companies by finding which companies were mentioned together in the news, and aggregated the co-mentions per quarter. Companies were found using regular expressions that matched the writing style of news sources, and after this singled out by their company name and company ticker.Researchers have shown that linkages between entities and interconnectedness overall (such as contagion) often times significantly impact stock prices, which means that entities in networks are seen to be affected by events happening to other nodes in the network. We used this knowledge and RiskRank to extract company level risk that spreads through the networks. The output we got was a signal between 0 and 1 for each company for each aggregated period. In our case, once for each quarter.
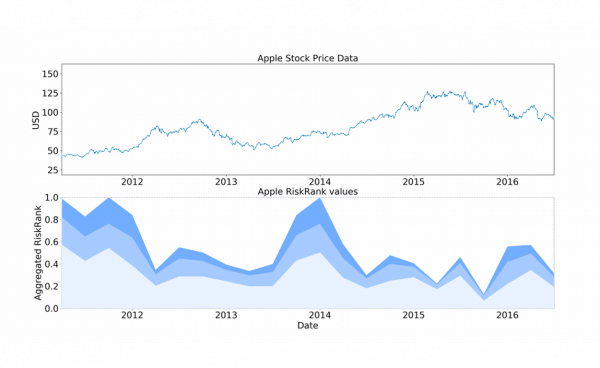
Figure 2: Sentiment risk extracted for Apple Inc. using our approach over the period 2011 – 2016
Finally, we analysed how company stock prices behaved at different risk levels over a five-year period (see Figure 2). We found that companies that reached the maximum level of news-sentiment risk were up to 13 percentage points more likely to have stock price decline one month later.
If you would like to know more about how we build custom-made AI solutions for the financial sector, get in touch.
About
Join the 5000+ subscribers who read the Silo AI monthly newsletter to be among the first to hear about the latest insights, articles, podcast episodes, webinars, and more.