The era of information
We live in a time period characterised by the shift from traditional industries to an economy based on information technology. Thanks to this, companies are more aware every day of the value of the information they accumulate in a seemingly natural way.This transformation does not come without its own difficulties. For example, every year the amount of information generated grows exponentially. Ninety per cent of the data in the world today has been created in the last two years alone. Our current output of data is roughly 2.5 quintillion bytes a day – that is, 25 followed by 17 zeros, every single day. As the world steadily becomes more connected with an ever-increasing number of electronic devices, that amount of daily created data is only set to grow over the coming years. When confronted with these staggering amounts of information, any team of human analysts would be rightfully overwhelmed. So what can companies do in order to make use of their valuable information?A recurring problem that is becoming more and more common is how to determine the interest of customers in different contexts. Whenever a company wants to sell high volumes of particular products to specific audiences, it should take care to focus its efforts (e.g. marketing) on the appropriate group of customers. This is not straightforward, which becomes more evident when considering the number of users and products (both often in the hundreds of thousands or even millions) and the different interactions they may have. For example, how is it possible to know what items are interesting for a new user or how to recommend products when there are no ratings available?In the past, very general and simplistic strategies were used based on factors like gender, age or geographical location. However, those rules fail to capture the value of the extensive information available about products and customers. It is not reasonable to expect humans to go through every single combination, something that would probably take years. So, once more the question stands: what to do then?
Enter machine learning
Machine learning refers to giving computers the ability to learn without being explicitly programmed. This sounds particularly convenient for our recommendation problem, and indeed machine learning has become the heart and soul of recommender systems all over the world. The most relevant technology companies all use machine learning powered recommendation systems with huge success. As a snapshot of how relevant these systems have become, consider that:
- At Netflix, two-thirds of the movies watched are recommended.
- At Google, news recommendations improved click-through rate (CTR) by 38%.
- For Amazon, 35% of sales come from recommendations.
Systems of this type are commonplace, even if users are unaware of them. Recommender systems are used for giving advice on songs, suggesting books, finding clothes to buy, and so on, and are important also in social networking and the entertainment industry, as in general they improve the ability of customers to make choices.
The diagram below gives an overview of recommender systems.
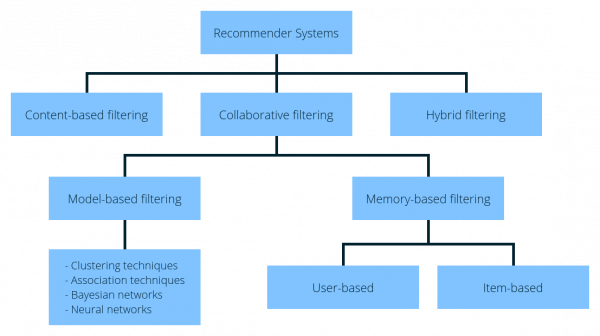
- Content-based filtering: many features are identified for each of the products. After this, the problem can be approached with standard machine learning methods. The challenge lies in the effort needed to extract all those features for every product.
- Collaborative filtering: this is based on the relationship between users and items. Conveniently, no specific information about users or items is strictly required. Instead, some kind of score for user–item interactions is needed (e.g. rankings or just purchases).
- Model-based filtering: this is based on matrix factorisation. It can deal better with scalability and sparsity.
- Memory-based filtering: this can use similar products (i.e. ‘users who liked this item also liked …’) or similar users (‘users who are similar to you also liked …’) to produce recommendations.
Let’s step back for a moment and consider once more the magnitude of the task at hand. Imagine, for instance, a retail store that sells hundreds of thousands of different products. The customers for this store are in the millions, and the company wants to create a system to recommend specific products to specific users. With millions and millions of user–product interactions, finding patterns is a truly daunting task … for humans. Other than determining general market segments (e.g. sex, age, location), it is difficult to determine what to offer and to whom. Furthermore, to do this, specific user information and a certain level of knowledge about each product must be available.
Concluding remarks
To conclude, it is important to highlight that recommender systems can be (and indeed are) already used in a surprisingly broad array of fields beside the retail market and the books/movies environment. Basically, any type of organisation trying to do, for example, any of the following would benefit from a recommender system: offer personalised assistance to its customers to make a product selection; know in which of its products particular individuals or market segments are more likely to be interested (e.g. to focus its marketing); draw data-driven relationships between customers (e.g. to infer non-obvious market segments) or products (e.g. to determine what products are more likely to be bought together).Considering the scenario depicted above, it should be obvious that many organisations can benefit from recommender systems. From travel agencies recommending travel destinations or hosting locations to dating sites determining appropriate matches; from anyone interested in developing truly personalised marketing to those looking forward to offer the right option to their customers. If a business is not using a recommender system yet, the question is most likely not whether it can benefit from one, but why is it not using one already.
Work in retail? We have published a White Paper on 'AI in retail – Fraud detection online & offline'. Get your free copy.
About
Join the 5000+ subscribers who read the Silo AI monthly newsletter to be among the first to hear about the latest insights, articles, podcast episodes, webinars, and more.