Predictive maintenance is the key in ensuring your operations run smoothly, as you get to spot the upcoming failures before they happen. Companies today have the opportunity to leverage intelligent predictions and alerts that ensure their equipment is performing as it should. Catching the maintenance needs in advance decreases the downtime, increases savings, and lets companies plan ahead.
Machine learning for predictive maintenance can provide a detailed risk assessment of potential failure events, and highlight situations with elevated risk. Combine it with a network of IoT sensors in your mission-critical equipment and 5G connectivity, and you’ll be on your way towards more reliable operations.
In this article, we’ll discuss with Emil Eirola, our Senior AI Scientist, about machine learning for predictive maintenance.
You’ll learn about:
- How to embed machine learning into your product development: what kind of data the product collects, processes and sends forward
- The benefits of edge computing: what can be calculated in edge, and what should be transferred to the cloud
- How to get a head start
- How can you ensure that you have the right data available
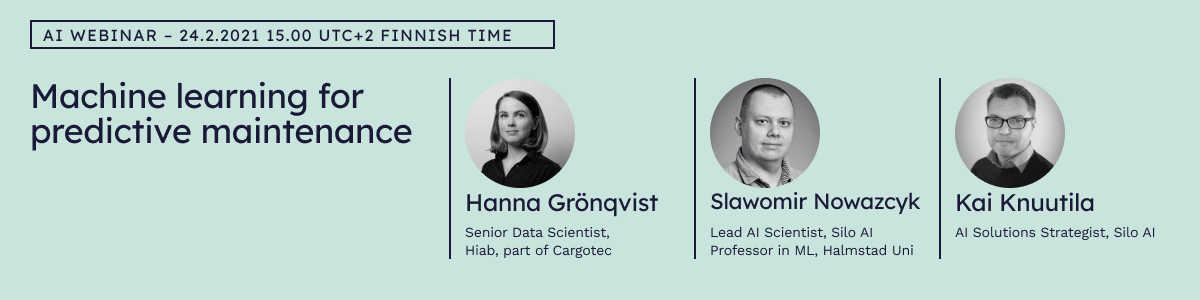
Roots of predictive maintenance starts with embedding machine learning into your product R&D
Features allowing predictive maintenance should be an integral part of your product. Therefore its introduction should start already in the product development phase. Using machine learning for predictive maintenance may produce obvious gains such as competitive edge and credibility in the market.
Silo AI's AI Scientist Emil Eirola believes embedding machine learning starts with understanding the problem. In his view, there should be a clear definition of the problems the machine learning model is expected to solve:
“When building machine learning for predictive maintenance, it is important to determine whether you will focus on remaining useful life or risk of failures. Alternatively, an anomaly detection model could be designed to flag any types of irregular activity, which can help detect rare types of failures early.”
When training the machine learning model for predictive maintenance, training data is needed for both normal and error states.
When training the machine learning model for predictive maintenance, training data is needed for both normal and error states. Therefore you’ll need extensive data covering the full range of normal operations, as well as examples of exceptional cases that the model would learn to flag early.
"The nature of the failure events also matters – whether they are generally sudden, or a result of a gradual degradation." Emil adds.
The benefits of edge computing
Today, it is possible to combine the best of edge and cloud computing, in order to create an efficient network of data and analytics flow. Edge computing means calculating and analyzing the data as close to its source as possible: often, close to the sensor collecting the data itself.
There are several reasons why edge computing can be practical for companies to invest in. Often, the amount of the collected data is huge, which leads to considerations of speed. In addition, there can exist legislative or security and compliance reasons that restrict moving the data offsite.
In other situations, the challenges result from the lack of reliable and fast connectivity. Sometimes moving the data to the cloud may just be too slow, if the output is needed within milliseconds.
"In these cases, edge computing can offer a great way to be able to make predictions for detecting local failure events. In areas of poor connectivity, the combination of edge and cloud usually allows the most sophisticated AI solutions." Emil says.
Cloud computing is still needed. All calculations that require combining raw data from several edge nodes, will need to be gathered together somewhere. With the combination of edge and cloud, optimized data points can be transferred more quickly to cloud. In the cloud, there’s access to more computing power and larger storage.
“It is likely that the model can achieve the best accuracy when having access to as much of the raw data as possible. An example of this would be identifying discrepancies between the performance of different devices.”, Emil comments.
Introducing machine learning for predictive maintenance into existing equipment
There’s always a possibility of retrofitting existing equipment by adding various sensors to collect data. Once you are collecting data, you can add and integrate machine learning models to work with that data.
However, whatever you bring to the device itself after it’s been manufactured may not be as good as bringing those features already as part of product development. If you have predictive features as part of your product R&D, it’ll be much easier to build business models and new services to offer your clients around these capabilities.
Ensuring you have the right data available
As all machine learning solutions require data, it’s essential to get started with collecting it.
“Often it is helpful to talk to an AI Scientist as early as possible to understand what kind of data, and how much is needed to train the machine learning models.” Emil says.
Predictive maintenance is a smart and valuable way to improve your offering in the longer run.
When collecting the data, it is important to ensure that you have the right data and that you’re able to use it. Any legal, compliance or commercial contracts related issues should be investigated, such as who is owning the data: you as product manufacturer or your customer.
“More often than not, there is data available, but not necessarily a clear plan on how to use it. The best solution I see is to discuss with an expert who can help you assess what you currently have, whether you can use it, and what you still need more of. If your vision is to have business models and services around your products and their good maintenance, machine learning for predictive maintenance is a smart and valuable way to improve your offering in the longer run.” Emil concludes.

Want to dive deeper into machine learning for predictive maintenance?
Join our upcoming webinar, machine learning for predictive maintenance – sign up here.
Get in touch with Business Development Executive Roope Suomalainen, roope.suomalainen@silo.ai or on Linkedin.
About
Join the 5000+ subscribers who read the Silo AI monthly newsletter to be among the first to hear about the latest insights, articles, podcast episodes, webinars, and more.