Our Data Privacy Expert Erlin Gulbenkoglu took the stage on the International Women's Day at Women in Data Science event to talk about how to build transparent AI systems. Previously in this blog she has discussed the GDPR & Privacy by Design in AI as well as hosted a technical webinar on model interpretability. Here’s a quick recap of her presentation.Starting off by looking at the Draft Ethics guidelines for trustworthy AI (EU) and the GDPR principles from the transparency perspective, Erlin set up a framework on how to think about transparency. The EU Ethics principles are presented below.
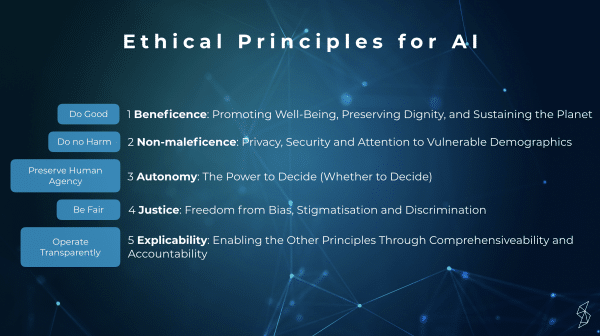
From these ethical principles the third ‘Preserve Human Agency’ and ‘Operate Transparently’ have clear connections with building transparent artificial intelligence. These two are very similar to the GDPR articles 22 and 13, that focus on:Article 22: Right not to be subject to a decision based ‘solely’ on automated processing.Article 13: Information on the logic involved should be told to the data subjects.With this framework in mind, the presentation focused on the following question:
Why transparency matters?
1) In order to have control over the system people need to understand it.
Comprehending of AI systems shouldn’t be limited to those that develop the models or are otherwise more inclined to understand the system (e.g. mathematicians, software developers). Everyone should be able to have control and to do this they need information on how the system works.
2) To measure accountability.
When something goes wrong, will humans blame the AI system or the company that built it? If you have the explicability component in place, that helps you to measure the accountability of AI.
3) When we explain a prediction sufficiently, a user can take some action based on it.
When people understand how the system works, people are happier to use it since they can trust it more. This trust comes from their ability to take action based on the explanation. For instance, if a self-driving car made a mistake, it would be understood why that happened, and in the best scenario, the responsible person/company could be found. Also if would help to prevent such a mistake in the future.
4) To be able to explain machine learning based decisions improves transparency and acceptance of complex models.
Let’s take an example like the insurance industry. If many claim decisions will be based on AI in the future, people will still need to be able to understand how those decisions were made. People will also be able to complain and get justice if something went wrong.
5) To assess a model in detail, for example, the developer can evaluate the model and identify the failure modes.
Working on different explainability methods, such as SHAP or GradCAM can be helpful in creating a better model, because you will further understand what is happening and why certain decisions are made.
To find out more on how Silo.AI builds AI models that you can trust, read about our Solutions or get in touch.
About
Join the 5000+ subscribers who read the Silo AI monthly newsletter to be among the first to hear about the latest insights, articles, podcast episodes, webinars, and more.